Immersive Question-Directed Visual Attention
Ming Jiang*, Shi Chen*, Jinhui Yang, Qi Zhao
University of Minnesota
While most visual attention studies focus on bottom-up attention with restricted field-of-view, real-life situations are filled with embodied vision tasks. The role of attention is more significant in the latter due to the information overload, and attention to the most important regions is critical to the success of tasks. The effects of visual attention on task performance in this context have also been widely ignored. This research addresses a number of challenges to bridge this research gap, on both the data and model aspects.
Specifically, we introduce the first dataset of top-down attention in immersive scenes. The Immersive Question-directed Visual Attention (IQVA) dataset features visual attention and corresponding task performance (i.e., answer correctness). It consists of 975 questions and answers collected from people viewing 360° videos in a head-mounted display. Analyses of the data demonstrate a significant correlation between people's task performance and their eye movements, suggesting the role of attention in task performance. With that, a neural network is developed to encode the differences of correct and incorrect attention and jointly predict the two. The proposed attention model for the first time takes into account answer correctness, whose outputs naturally distinguish important regions from distractions. This study with new data and features may enable new tasks that leverage attention and answer correctness, and inspire new research that reveals the process behind decision making in performing various tasks.
Dataset
Featuring task-driven attention in immersive viewing of 360° videos, our IQVA dataset contains 975 YouTube video clips in 4K equirectangular format. We offer questions, answers and ground-truth eye-tracking data of 14 participants each. The data are grouped based on the correctness of the participants' answers.
The examples below show typical attention patterns leading to correct and incorrect answers. Spatio-temporal fixation maps overlaid as contours, and the most salient regions are highlighted.
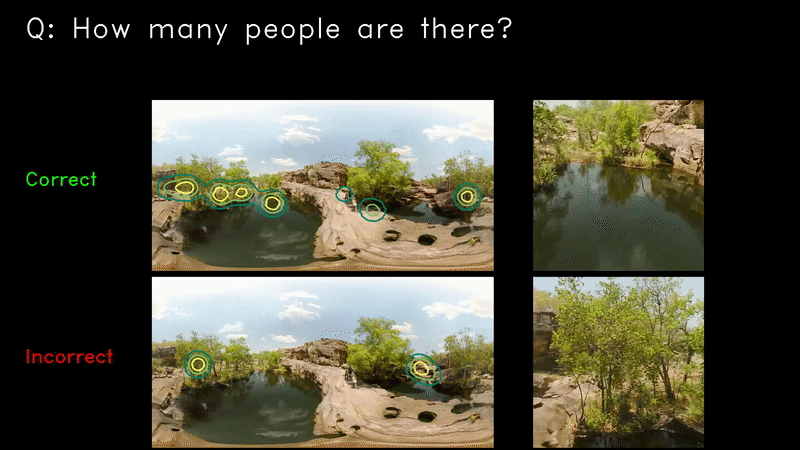
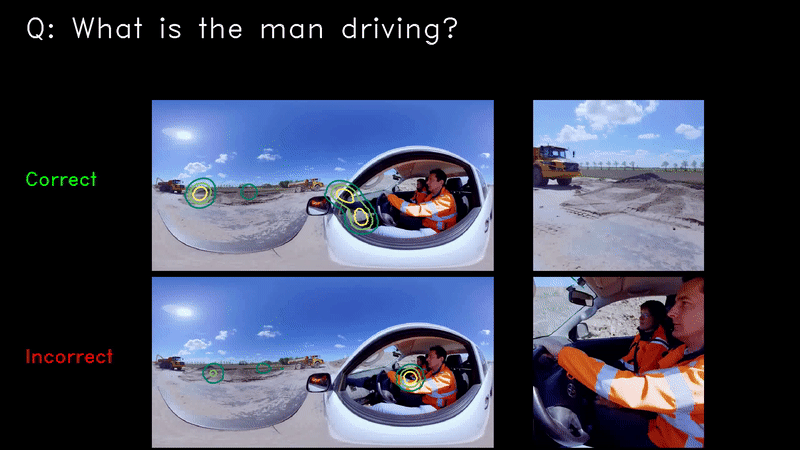
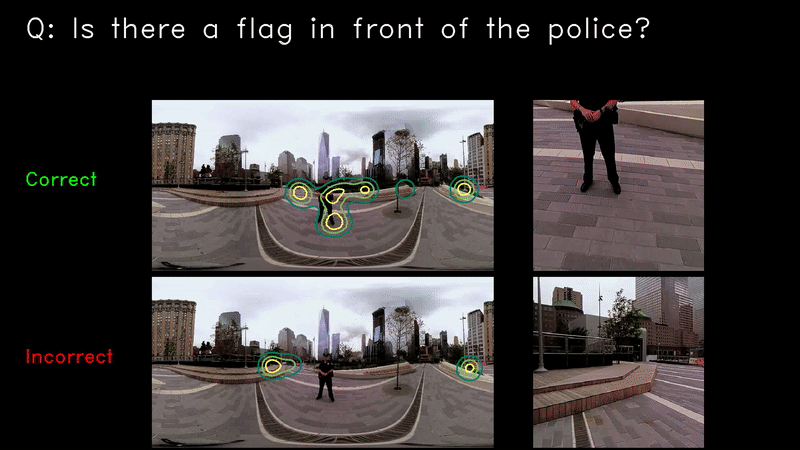
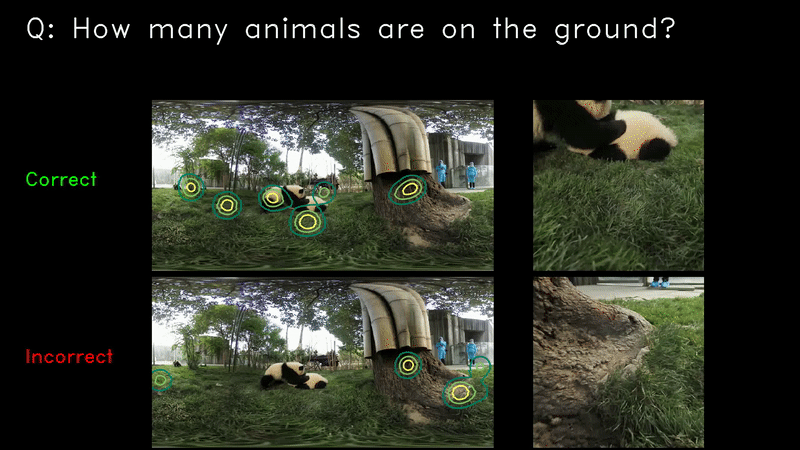
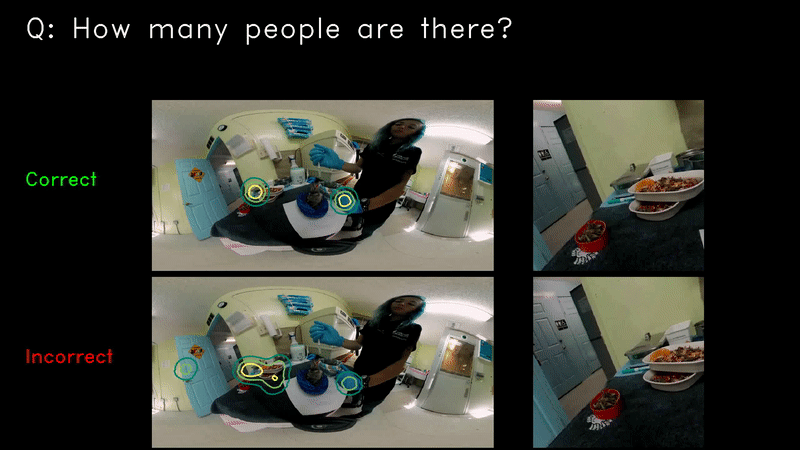
Correctness-Aware Attention Prediction
We propose a novel correctness-aware attention prediction network to addresses both issues. Different from conventional gaze prediction models, our model simultaneously computes correct and incorrect attentions and enables knowledge sharing among them. We use a new fine-grained difference (FGD) loss to better differentiate the two types of attention. Results are shown below:
Model | Correct | Incorrect | ||||||||
---|---|---|---|---|---|---|---|---|---|---|
CC | NSS | KLD | SIM | sAUC | CC | NSS | KLD | SIM | sAUC | |
SALICON | 0.407 | 2.010 | 1.645 | 0.350 | 0.429 | 0.389 | 1.914 | 1.689 | 0.326 | 0.431 |
SALNet | 0.412 | 2.028 | 1.560 | 0.347 | 0.451 | 0.380 | 1.946 | 1.703 | 0.329 | 0.397 |
ACLNet | 0.402 | 1.938 | 1.606 | 0.341 | 0.448 | 0.378 | 1.900 | 1.717 | 0.322 | 0.424 |
Spherical U-Net | 0.268 | 1.225 | 1.955 | 0.262 | 0.333 | 0.247 | 1.167 | 2.085 | 0.234 | 0.343 |
Multi-Att | 0.426 | 2.293 | 1.479 | 0.365 | 0.446 | 0.411 | 2.225 | 1.570 | 0.344 | 0.447 |
Multi-Att + SWM | 0.439 | 2.316 | 1.434 | 0.368 | 0.456 | 0.422 | 2.205 | 1.561 | 0.344 | 0.455 |
Multi-Att + SWM + FGD | 0.441 | 2.375 | 1.429 | 0.371 | 0.462 | 0.424 | 2.267 | 1.524 | 0.345 | 0.469 |
Download Links
Please cite the following paper if you use our dataset or code:
@inproceedings{jiang2020fantastic,
title={Fantastic Answers and Where to Find Them: Immersive Question-Directed Visual Attention},
author={Jiang, Ming and Chen, Shi and Yang, Jinhui and Zhao, Qi},
booktitle={Proceedings of the IEEE/CVF Conference on Computer Vision and Pattern Recognition},
pages={2980--2989},
year={2020}
}
Acknowledgment
This work is supported by National Science Foundation grants 1908711 and 1849107.