VisualHow: Multimodal Problem Solving
Jinhui Yang*, Xianyu Chen*, Ming Jiang, Shi Chen, Louis Wang, Qi Zhao
(* Equal Contribution)
Department of Computer Science and Engineering
University of Minnesota
Recent progress in the interdisciplinary studies of computer vision (CV) and natural language processing (NLP) has enabled the development of intelligent systems that can describe what they see and answer questions accordingly. However, despite showing usefulness in performing these vision-language tasks, existing methods still struggle in understanding real-life problems (i.e., how to do something) and suggesting step-by-step guidance to solve them. With an overarching goal of developing intelligent systems to assist humans in various daily activities, we propose VisualHow, a free-form and open-ended research that focuses on understanding a real-life problem and deriving its solution by incorporating key components across multiple modalities. We develop a new dataset with 20,028 real-life problems and 102,933 steps that constitute their solutions, where each step consists of both a visual illustration and a textual description that guide the problem solving. To establish better understanding of problems and solutions, we also provide annotations of multimodal attention that localizes important components across modalities and solution graphs that encapsulate different steps in structured representations. These data and annotations enable a family of new vision-language tasks that solve real-life problems. Through extensive experiments with representative models, we demonstrate their effectiveness on training and testing models for the new tasks, and there is significant scope for improvement by learning effective attention mechanisms. Our dataset and models are available at https://github.com/formidify/VisualHow.
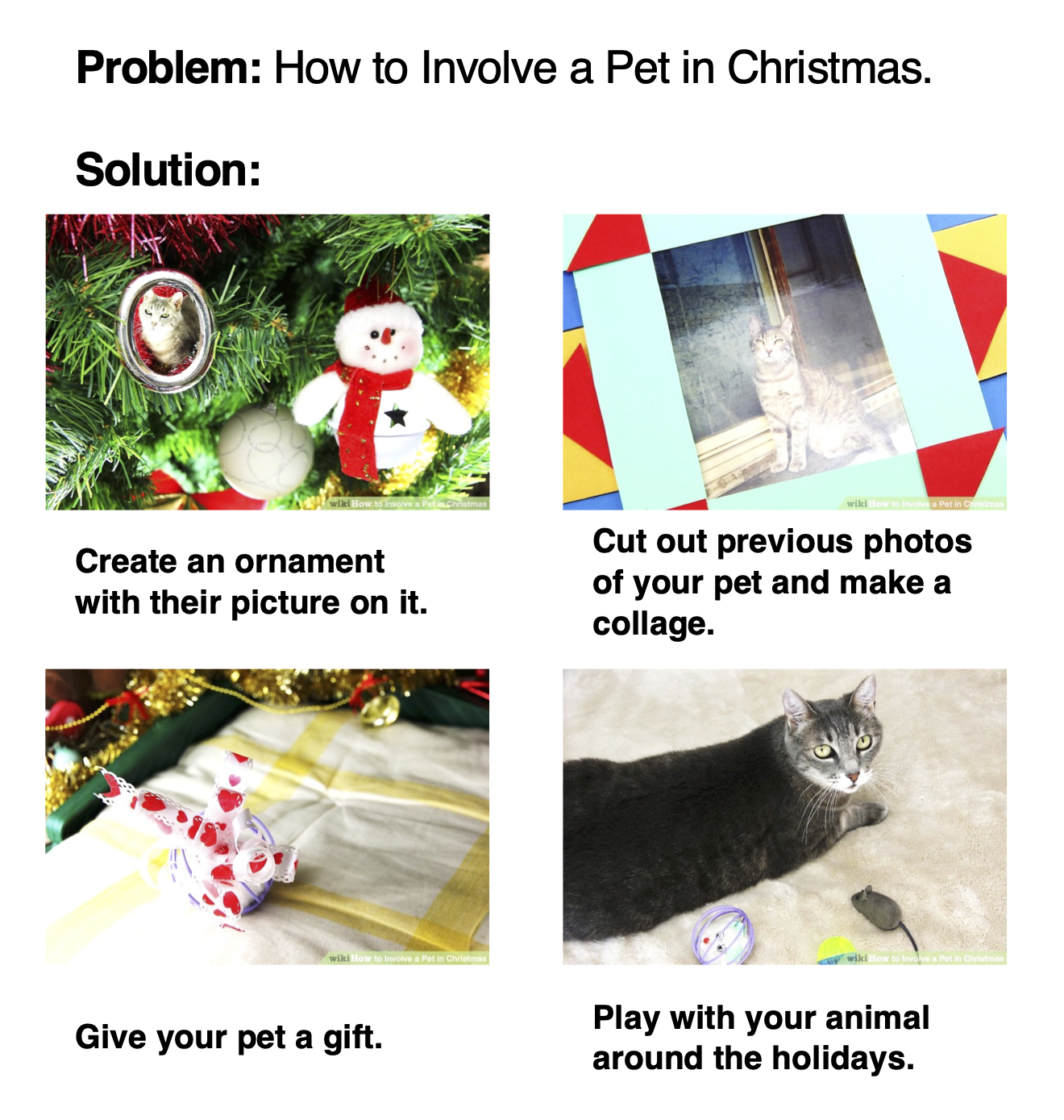
VisualHow is a vision-language task aiming to infer the solution to a real-life problem. The solution consists of multiple steps each described with an image and a caption.
Our work provides three novel contributions:
- A new VisualHow study aiming to provide the foundation for developing novel vision-language methods and pushing the boundaries of multimodal understanding of real-life problems and solutions;
- A new dataset that consists of diverse categories of problems, multimodal descriptions of solutions, and fine-grained annotations;
- Experiments on multiple new tasks on different aspects of the VisualHow problem and extensive analyses of various baseline models.
VisualHow Dataset
The VisualHow dataset consists of 18 categories of real-life problems and step-by-step solutions described with images and captions. The diversity and generality of problems and solutions also make VisualHow a more challenging dataset. In addition to the image-caption pairs, VisualHow provides annotations for solution graph and multimodal attention, which are essential for the understanding of problem-solution relationships and aligning the semantics between vision and language.
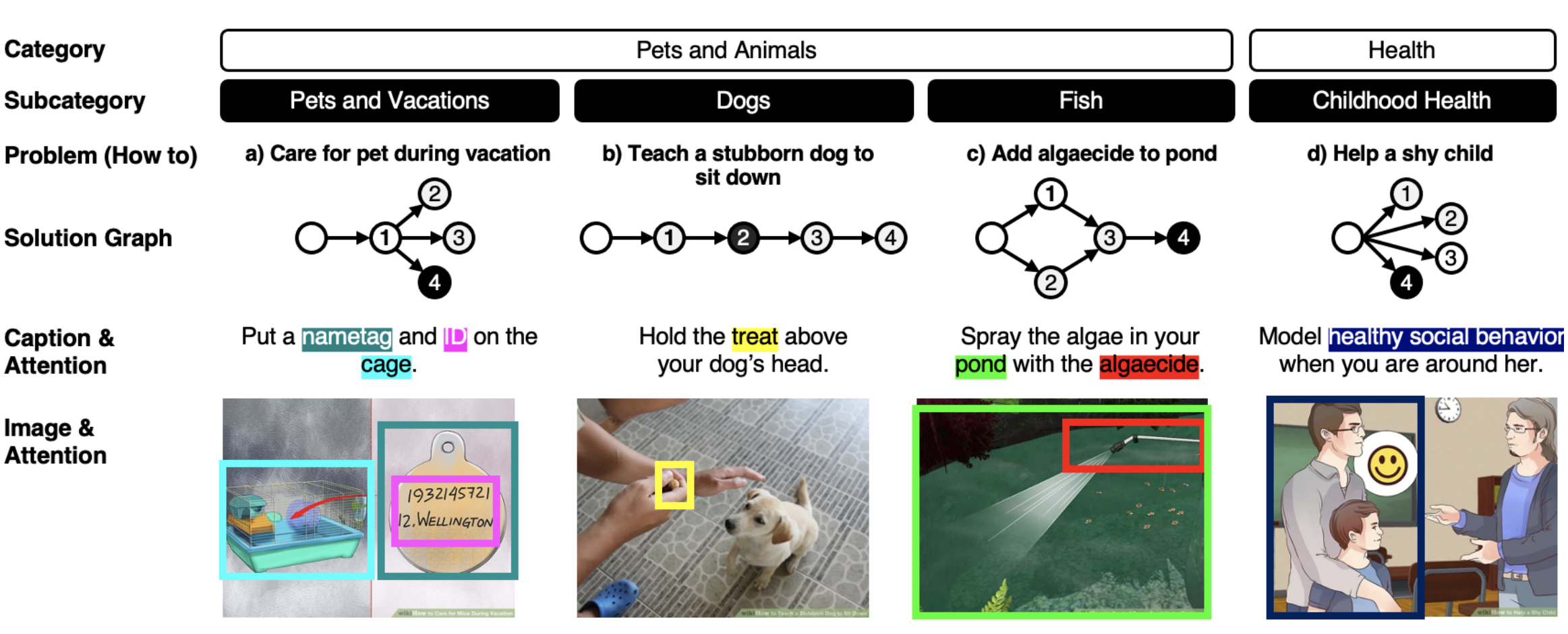
We provide a hierarchical structure that organizes our data into categories, sub-categories, problems, solution graphs, steps with image-caption pairs, and multimodal attention. Example steps are highlighted in the solution graph. Steps without a dependency are connected to an empty node.
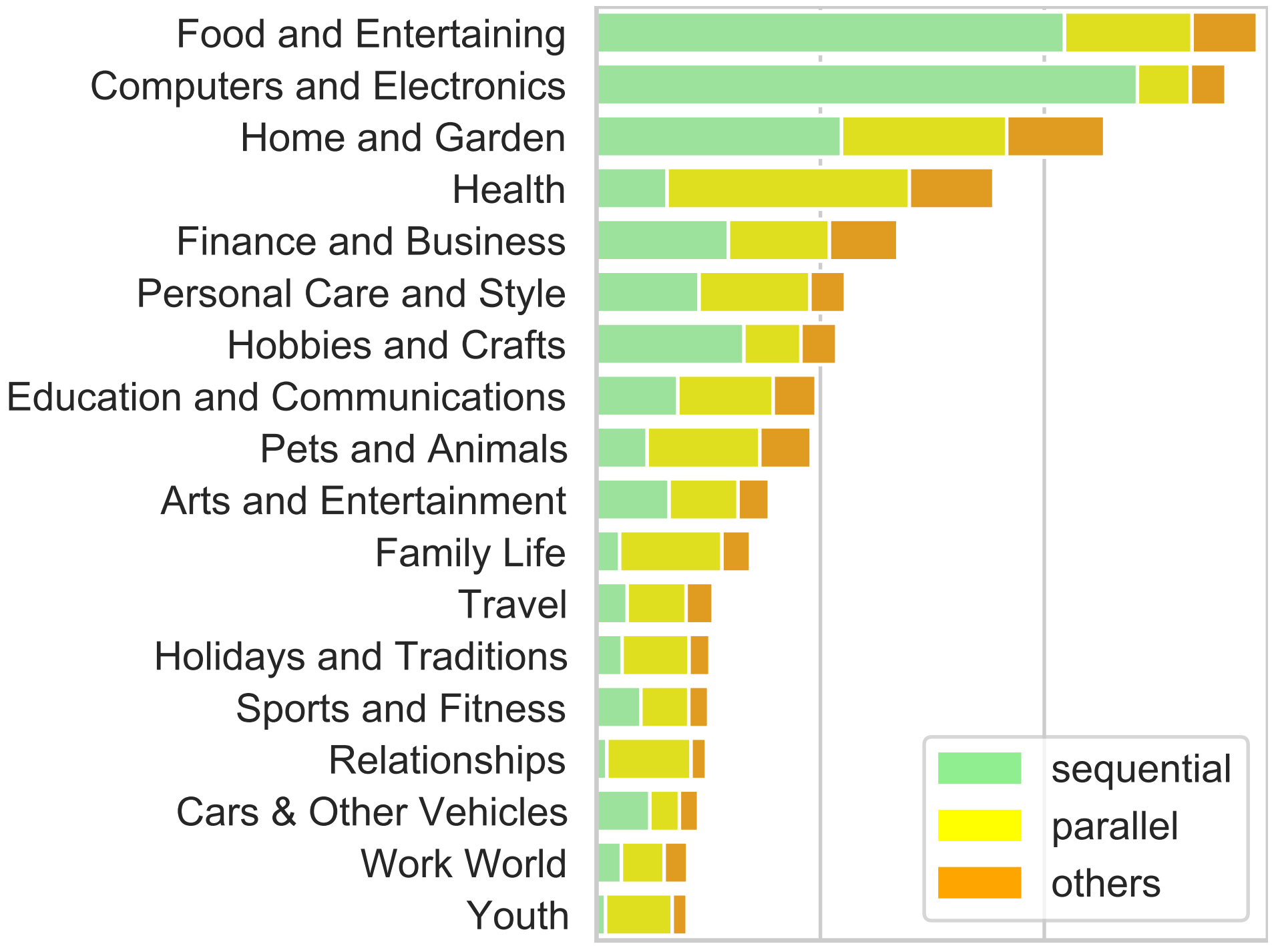
Number of problems in each category and the three types of solution graphs.
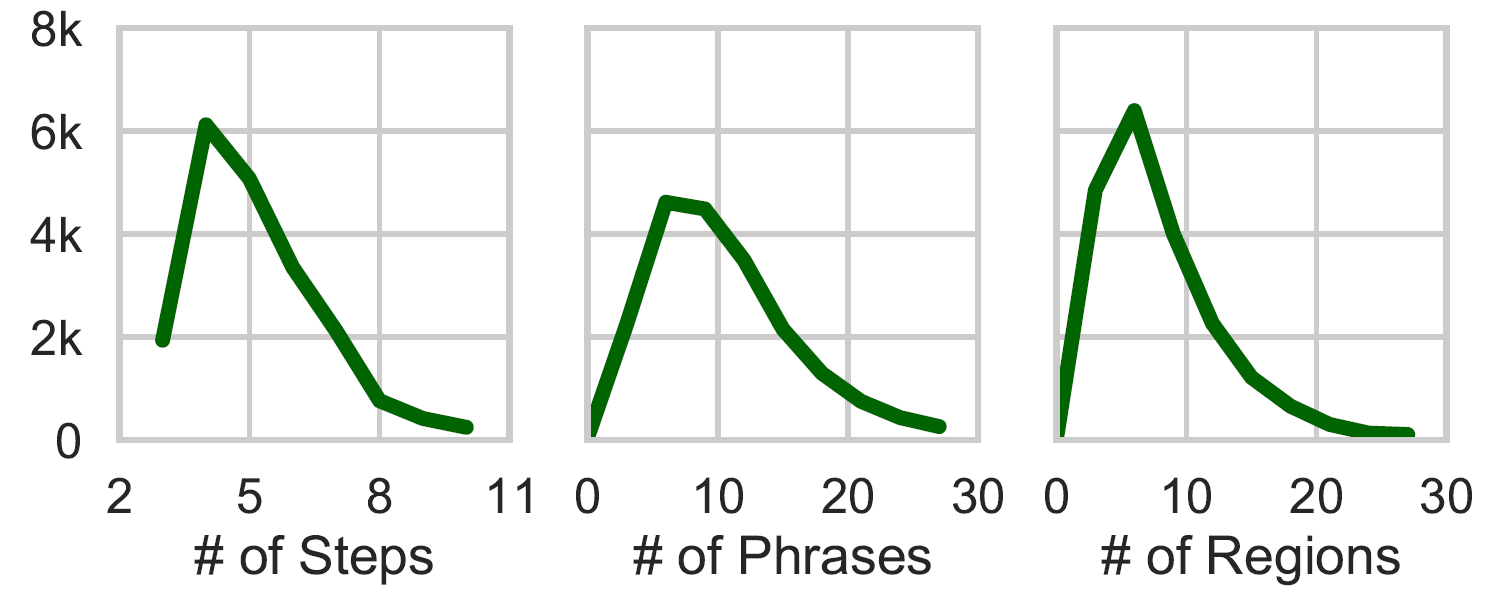
Distribution of solution steps and attention annotations.
VisualHow Tasks and Baseline Results
Our dataset enables new developments of intelligent problem-solving models that understand and generate solutions to real-life problems. As a first step towards this goal, we systematically analyze a series of baseline models that address new vision-language tasks. These tasks are Solution Steps Prediction, Solution Graph Prediction, Problem Description Generation, and Solution Caption Generation.
Solution Steps Prediction: Given the problem description and the previous solution steps, the models predict the image and caption of the next solution step by sorting two sets of candidate images and captions.
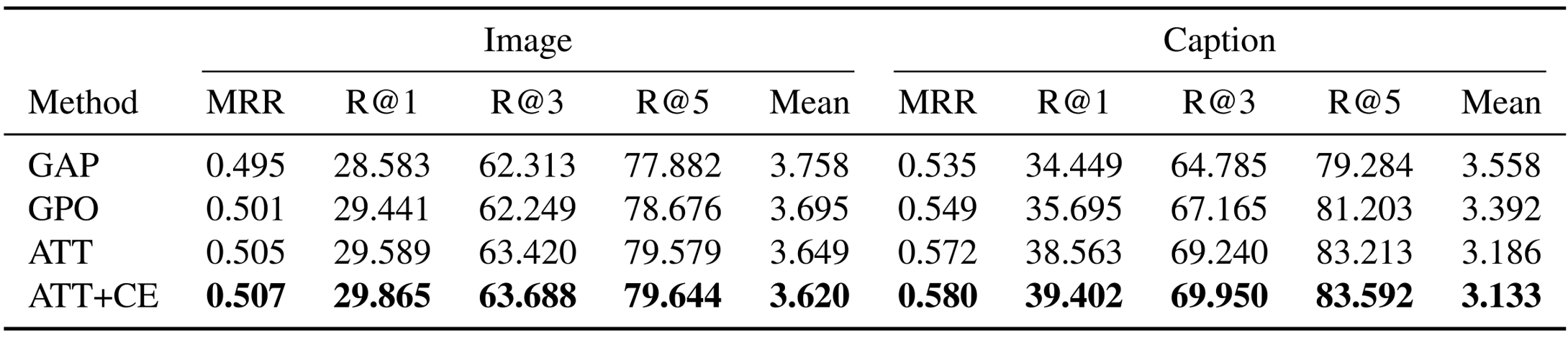
Solution Graph Prediction: Given problem and solution descriptions, the models predict the solution graph.
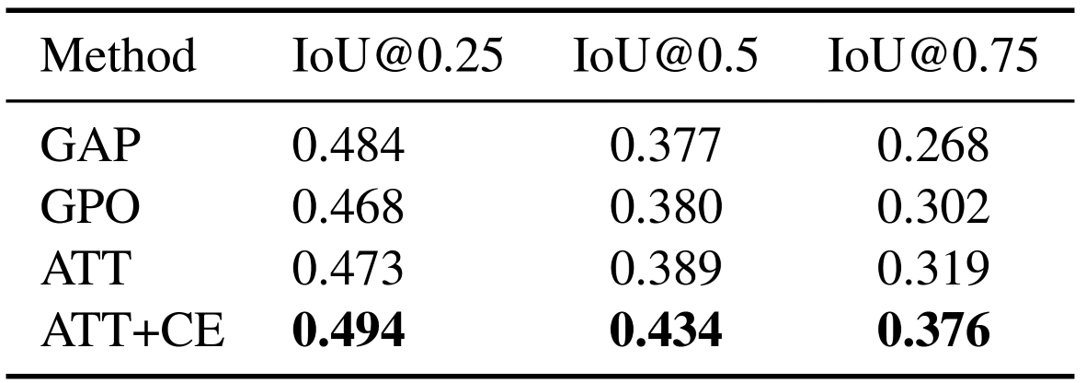
Problem Description Generation: Given the visual and textual solution descriptions, the models generate the problem description.
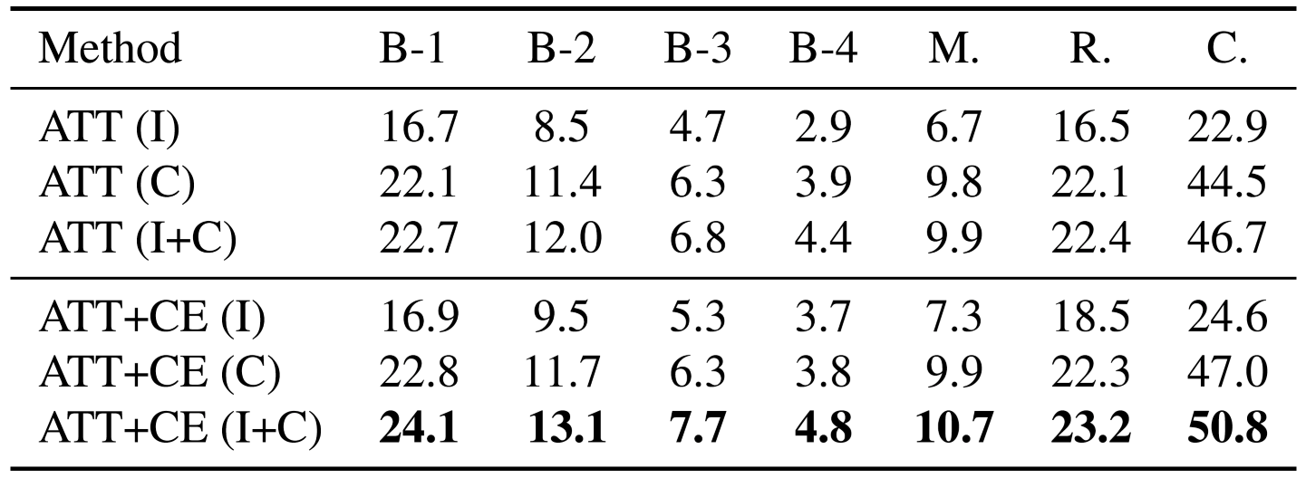
Solution Caption Generation:Given the problem description and solution images, the models generate solution captions.
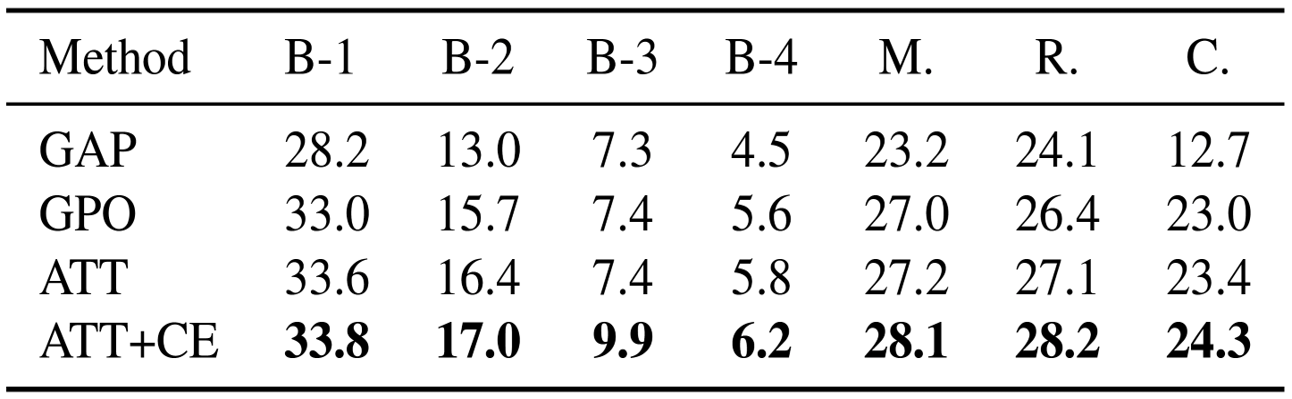
Download Links
Please cite the following paper if you use our dataset or code:
@InProceedings{visualhow2022,
author = {Yang, Jinhui and Chen, Xianyu and Jiang, Ming and Chen, Shi and Wang, Louis and Zhao, Qi},
title = {VisualHow: Multimodal Problem Solving},
booktitle = {CVPR},
year = {2022}
}
Acknowledgment
This work is supported by National Science Foundation grants 1908711.