AiR: Attention with Reasoning Capability
Shi Chen*, Ming Jiang*, Jinhui Yang, Qi Zhao
University of Minnesota
While attention has been an increasingly popular component in deep neural networks to both interpret and boost performance of models, little work has examined how attention progresses to accomplish a task and whether it is reasonable. In this work, we propose an Attention with Reasoning capability (AiR) framework that uses attention to understand and improve the process leading to task outcomes. We first define an evaluation metric based on a sequence of atomic reasoning operations, enabling quantitative measurement of attention that considers the reasoning process. We then collect human eye-tracking and answer correctness data, and analyze various machine and human attentions on their reasoning capability and how they impact task performance. Furthermore, we propose a supervision method to jointly and progressively optimize attention, reasoning, and task performance so that models learn to look at regions of interests by following a reasoning process. We demonstrate the effectiveness of the proposed framework in analyzing and modeling attention with better reasoning capability and task performance. The code and data are available at https://github.com/szzexpoi/AiR.

Attention is an essential mechanism that affects task performances in visual question answering. Eye fixation maps of humans suggest that people who answer correctly look at the most relevant ROIs in the reasoning process (i.e., jeans, girl, and bag), while incorrect answers are caused by misdirected attention.
Our Attention with Reasoning capability (AiR) study provides three novel contributions:
- AiR-E: a new quantitative evaluation metric to measure attention in the reasoning context, based on a set of constructed atomic reasoning operations.
- AiR-M:a supervision method to progressively optimize attention throughout the entire reasoning process.
- AiR-D: an eye-tracking dataset featuring high-quality attention and reasoning labels as well as ground truth answer correctness.
Reasoning-Aware Attention Supervision
We present typical examples to demonstrate that guiding models to look at places following the reasoning process (AiR-M) leads to better attention and task performance. While the compared state-of-the-art models only have a static attention map, AiR-M shows progressive attention following the reasoning process. Better alignments with the ground-truth are also shown (AiR-E scores).
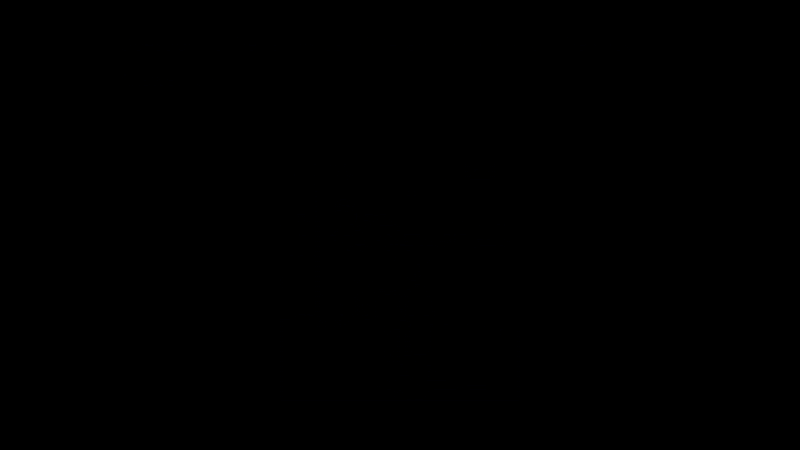
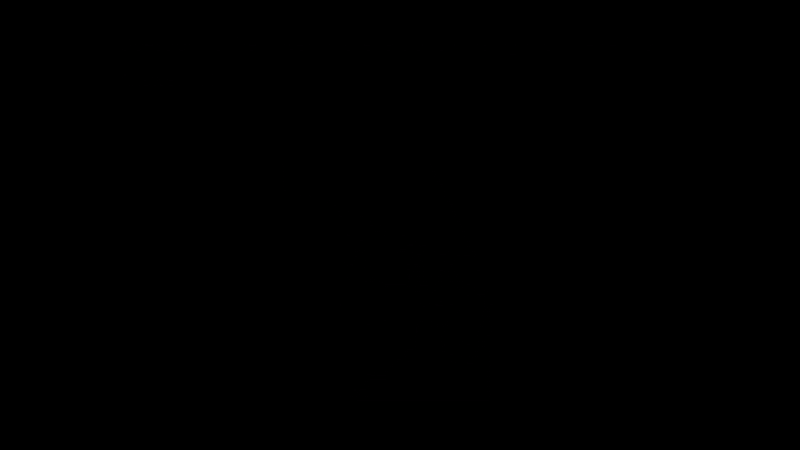
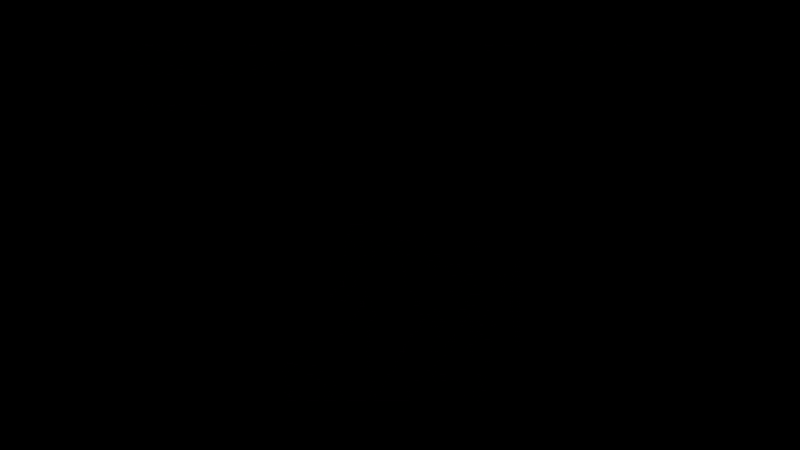
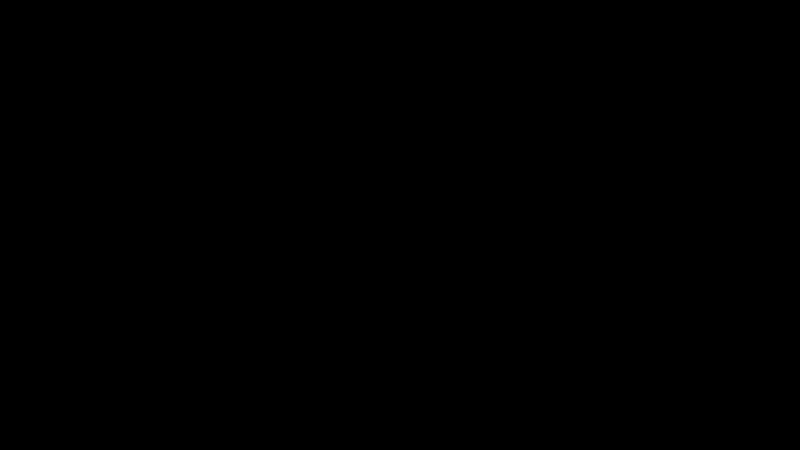
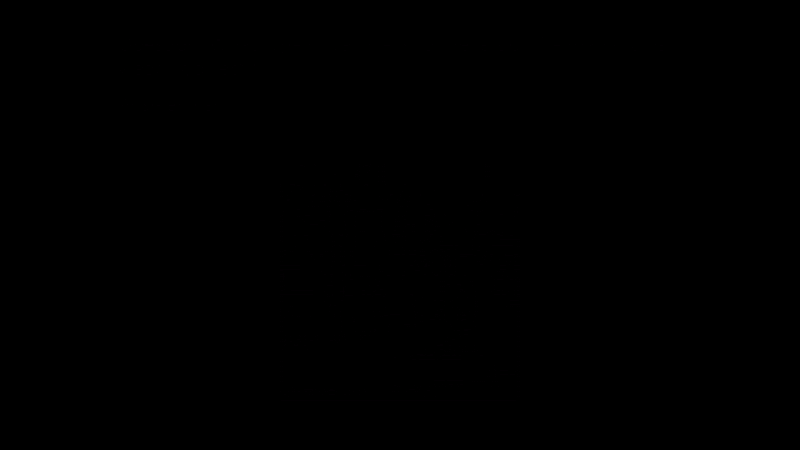
Eye-Tracking Data
We present typical examples to demonstrate that where humans look affect answer correctness. The correct and incorrect attention maps evolve over time, suggesting the temporal development of attention. The correct attention maps align better with reasoning annotations.
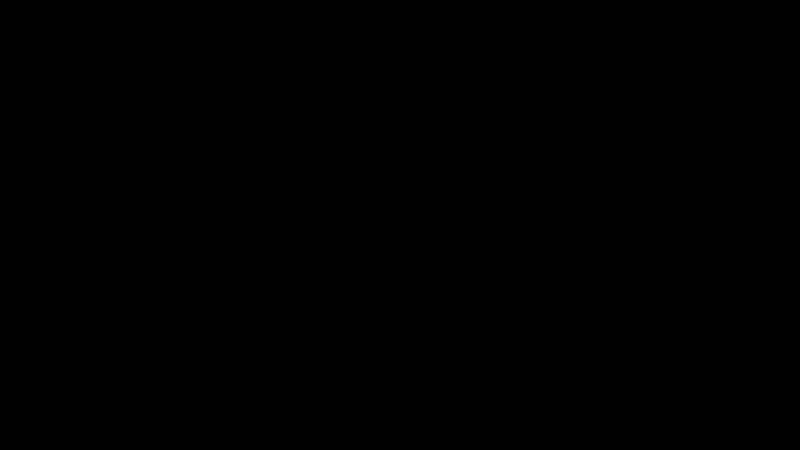
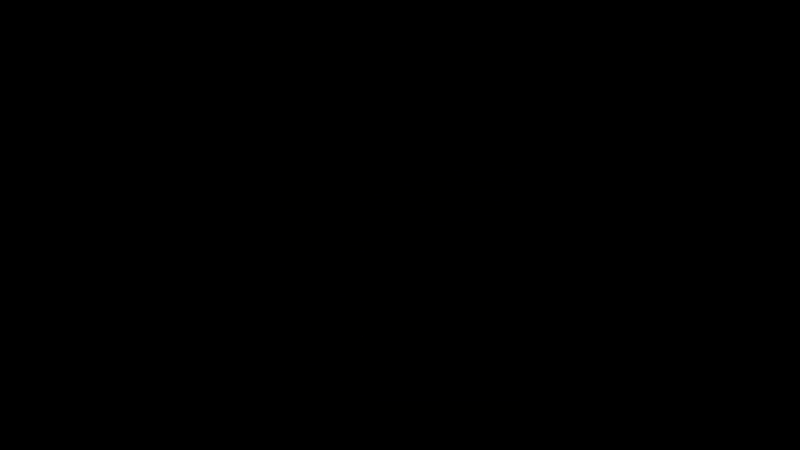
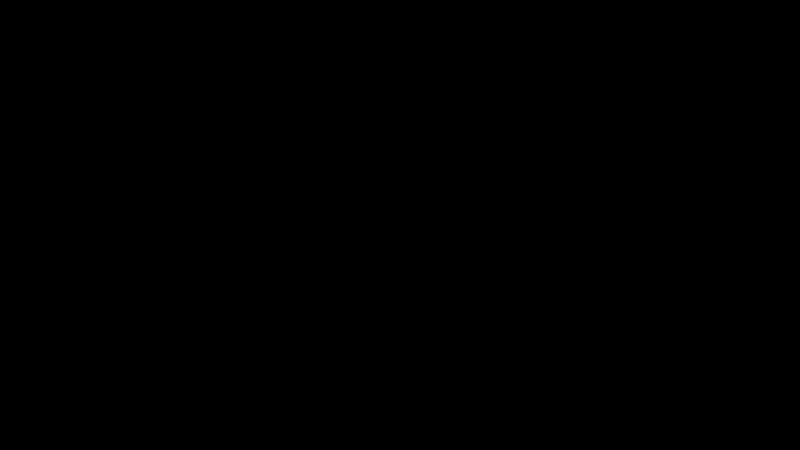
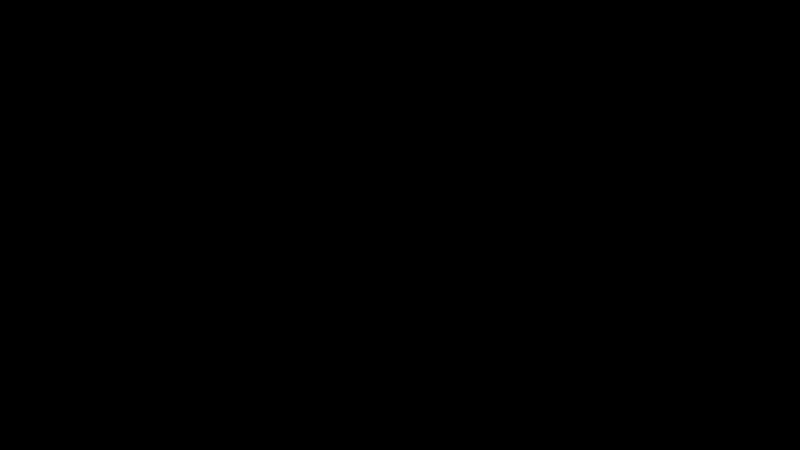
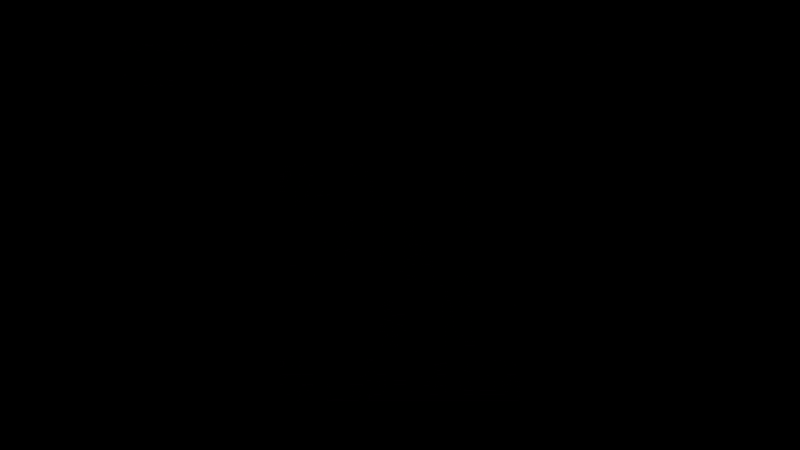
Download Links
Please cite the following paper if you use our dataset or code:
@InProceedings{air2020,
author = {Chen, Shi and Jiang, Ming and Yang, Jinhui and Zhao, Qi},
title = {{AiR}: Attention with Reasoning Capability},
booktitle = {European Conference on Computer Vision (ECCV)},
year={2020}
}
Acknowledgment
This work is supported by National Science Foundation grants 1908711 and 1849107.