Protein-protein interactions
Sequencing of the genomes of several species (including humans) opens the door to a new understanding of biological and cellular function, as well as to the elucidation of possible genetic mechanisms of many diseases, or to their cure through genetic manipulation. Current emphasis is not on the chemical structure and properties of individual molecules, but rather on the development of large-scale strategies to determine gene function and expression. Understanding the relationship between gene expression data and the underlying genomic sequence is crucial to understanding the machinery of living cells.
Large-scale gene sequencing projects are quickly producing vast amounts of sequence data, whereas three dimensional protein structures are available only for a small fraction of known protein sequences, with the gap increasing rapidly. The three dimensional structure of a protein generally provides more information about its function than the underlying amino acid sequence. The best methodology to determine the three dimensional structure of a protein is by X-Ray diffraction or NMR spectroscopic studies. However, both techniques are time consuming. It is the goal of structural genomics to aid in the large-scale determination of three dimensional protein structure from sequence.
Various computational approaches for protein structure prediction exist that are based on different physical assumptions and that operate at different levels of description. At one extreme, we find homology modeling. Its intrinsic shortcoming is that it is limited to proteins whose sequences are sufficiently close to those of proteins of known structure. Despite recent progress to address this issue, when the sequence similarity falls below a 30% threshold, the quality of the models produced degrades. At the other extreme, ab initio methods have been introduced to treat proteins with no clear homologs, or with structures not yet observed experimentally. At their most fundamental level, first principles force fields and molecular dynamics simulation methods are used to predict protein structure. With current computing capabilities, it is possible to simulate relatively large proteins over small periods of time (of the order of nanoseconds, several orders of magnitude below the characteristic time scale of protein folding which is in the range of milliseconds to seconds). Although there has been substantial progress in recent years both in the determination of empirical force fields and on algorithmic development, further progress appears to be limited by the available computing resources. Furthermore, some of the force fields used are ultimately of empirical nature, and therefore it is not easy, a priori, to systematically improve on them (most notoriously terms that account for solvent mediated forces among residues). It is also possible that additional many body interactions are important in the folding problem, and such forces are difficult to model and parameterize.
To simulate larger proteins and longer times characteristic of protein
folding, simplified models of the protein were initially introduced.
A reduced poly peptide chain representation was considered by defining
two interaction centers per amino
acid. One atom of each residue was located on the position of the
Cα atom, whereas the second corresponded to the center of
mass of an averaged side group (shown in the figure are the bonds
connecting consecutive interaction centers. The molecule show is a
dimer, each monomer comprising two α helices connected by a
turn). At about the same time, the
concept of statistically derived force fields (or the corresponding
potentials) was introduced that makes no direct reference to physical forces.
With them, they described observed regularities in secondary
structure and included long range interactions between side chains.
A reduced model including these statistical
potentials succeeded in producing protein-like features, although
the structures obtained were of very low resolution.
The methodology has been further developed in recent years by using a
combination of physically motivated forces and statistically developed
potentials. It is currently believed that quantitative details of
protein structure can be studied in the reduced conformational space
of a lattice with such a simplified representation of the
protein and of the interaction forces.
It has long been recognized that hydrophobicity is the primary source of protein stability, and hydrophobicity mediated interactions are commonly believed to be dominant in the determination of protein structure, and protein-protein interactions. In spite of this observation, and of the fact that relatively detailed theories of hydrophobicity have been known for some time, little effort has been made to incorporate these theories into studies of protein structure and interaction. The main difficulty, of course, is related to the need to explicitly describe the solvent, and the concomitant increase in complexity in what already is a barely tractable problem. The current method of choice to circumvent this difficulty is the introduction of empirical ``buried surface area'' terms into the interaction energies to represent the effects of hydrophobicity. This implicit approach obviates the need to explicitly include the solvent in the calculation, and has allowed for impressive advances in the field of protein structure prediction. Computational tools based on this approximation are now routinely used in a large number of laboratories around the world.
Such an approach, however, cannot capture existing spatial and temporal correlations in the motion of distant residues that are physically mediated by the solvent. As a consequence, it has been found to be necessary to include ill defined or poorly understood long range or multibody potentials so as to provide for very basic features such as cooperativity in folding. Furthermore, very little is known about the effectiveness of modeling hydrophobicity as a surface area coverage term or a contact potential in thermodynamic and kinetic studies of either folding or protein-protein interactions. Unlike static studies of protein structure in which the desired outcome is the native configuration of the target protein, thermodynamic and kinetic studies require proper sampling of the energy landscape of the protein. This of course includes regions in which only a fraction of native contacts are present, and therefore the only meaningful interactions between some segments may be via the solvent.
We are developing a combined description of protein and solvent along the lines described above. Such a description could provide predictive, quantitative information of moderate resolution protein structure (around 3 Angstroms), as well as accurate estimates of thermodynamics quantities. The polypeptide is modeled as a chain of interaction centers on a lattice (Fig. 1 above), and the solvent is coarse grained into cubic cells of lateral size of the order of the thermal correlation length of water at ambient conditions (approximately 4 Angstroms). The density of the ith cell is decomposed into an average and a Gaussian flcutuation. Partial integration leads to an effective lattice gas (or discrete varible) description of local density fluctuations that is coupled to the instantaneous location of the interaction centers that correspond to the hydrophobic residues.
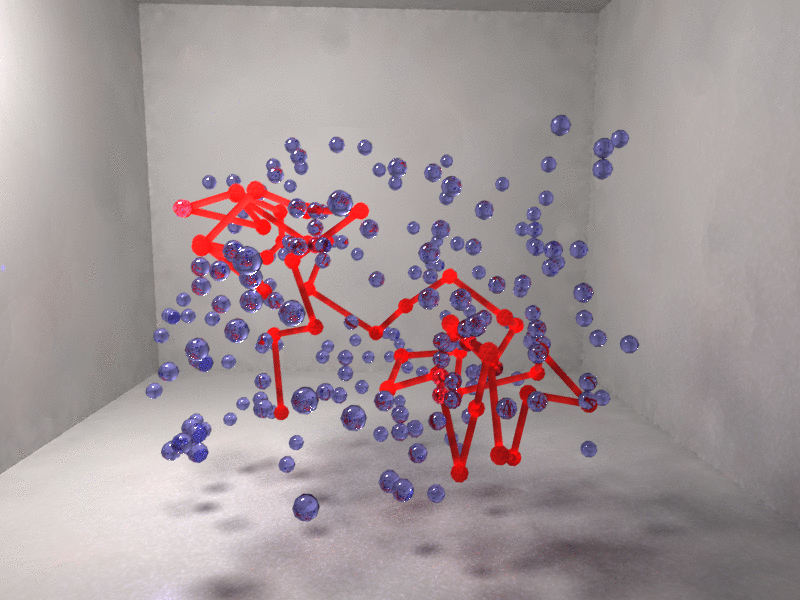
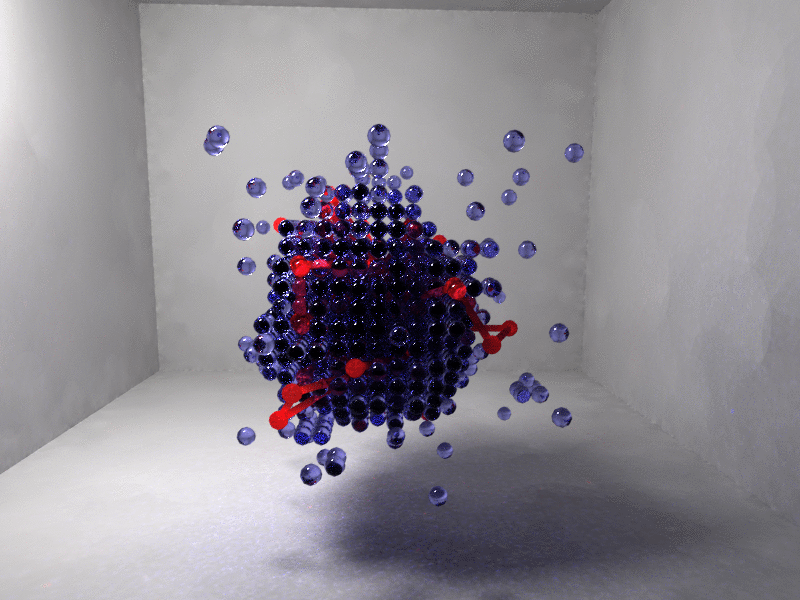
Figure 2. Interaction centers of the protein chain (red) and depleted or vapor cells (blue) are shown. Left, random coil with only isolated vapor fluctuations. Right, fully collapsed chain immersed in a vapor bubble.
Figure 2 shows the protein collapse for a model β-barrel synthetic polypetide with only a fraction of hydrophobic residues. Collapse is driven by a cooperative solvent fluctuation (blue indicates a region of depleted solvent) so that the hydrophobic residues are no longer exposed to the solvent.